If you find an error in what I’ve made, then fork, fix lectures/l04_mac.md, commit, push and create a pull request. That way, we use the global brain power most efficiently, and avoid multiple humans spending time on discovering the same error.
PDF
Attention Is All You Need

Neural Nets 3blue1brown
\[a_{l+1} = \sigma(W_l a_l + b_l)\]
A NN consists of addition, multiplication, and a non-linear function
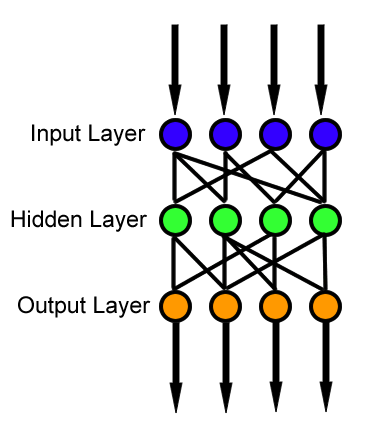
\[\mathbf{y} = \sigma\left(\begin{bmatrix}
w_{11} & w_{12} & \ldots & w_{1n} \\
w_{21} & w_{22} & \ldots & w_{2n} \\
\vdots & \vdots & \ddots & \vdots \\
w_{m1} & w_{m2} & \ldots & w_{mn}
\end{bmatrix}
\begin{bmatrix}
x_1 \\
x_2 \\
\vdots \\
x_n
\end{bmatrix} +
\begin{bmatrix}
b_1 \\
b_2 \\
\vdots \\
b_m
\end{bmatrix}\right)\]
\[{\mathrm{ OA}}_{(x,y,k)} = f \left ({\sum _{i=0}^{R-1} \sum _{j=0}^{S-1} \sum _{c=0}^{C-1} {\mathrm{ IA}}_{(x+i,y+j,c)} \times W_{(i,j,c,k)} }\right)\]
Assume N neurons
- N multiplications per neuron
- N + 1 additions per neuron
- 1 sigmoid per neuron
For efficient inference, additions and multiplications should be low power!

Kirchoff’s voltage law
The directed sum of the potential differences around any closed loop is zero
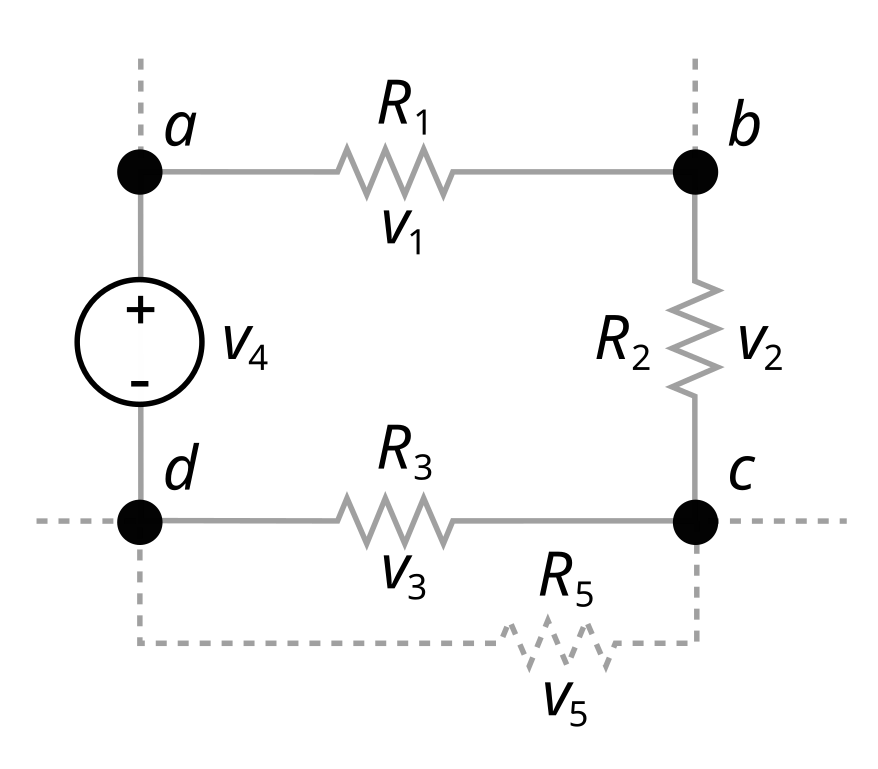
\[V_1 + V_2 + V_3 + V_4 = 0\]

Kirchoff’s current law
The algebraic sum of currents in a network of conductors meeting at a point is
zero
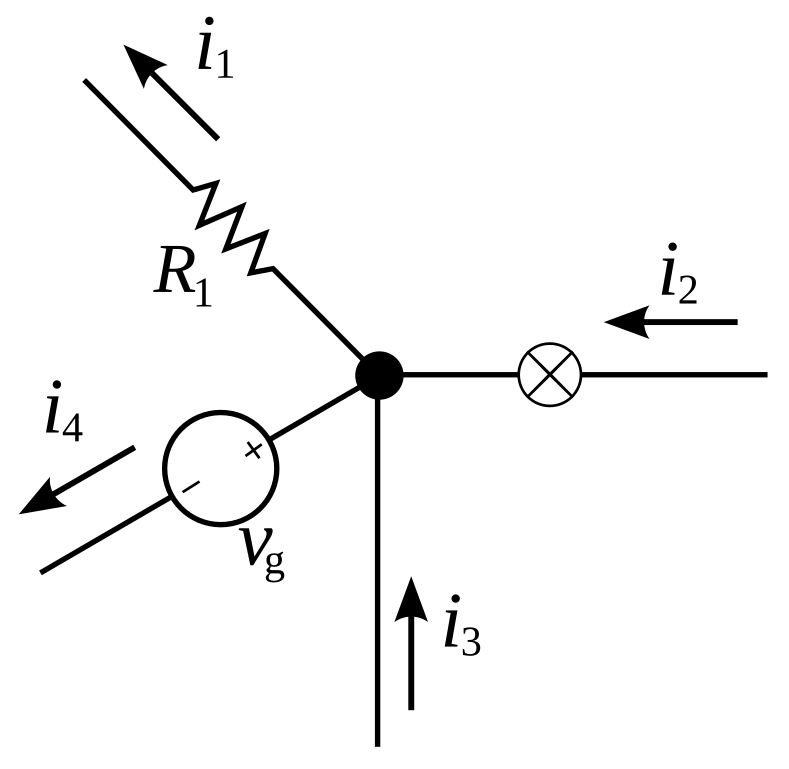
\[i_1 + i_2 + i_3 + i_4 = 0\]

Charge concervation
See Charge concervation on Wikipedia

\[Q_4 = Q_1 + Q_2 + Q_3\]
\[V_4 = \frac{ C_1 V_1 + C_2 V_2 + C_3 V_3}{C_1 + C_2 + C_3}\]
Multiplication
Digital capacitance
\[V_4 = \frac{ C_1 V_1 + C_2 V_2 + C_3 V_3}{C_1 + C_2 + C_3}\]
\[V_O = \frac{C_1}{C_{TOT}} V_1 + \dots + \frac{C_N}{C_{TOT}} V_N\]
Make capacitors digitally controlled, then
\[w_1 = \frac{C_1}{C_{TOT}}\]
Might have a slight problem with variable gain as a function of total capacitance
Mixing
\[I_{M1} = G_{m} V_{GS}\]
\[I_o = I_{M1} t_{input}\]

Translinear principle
MOSFET in sub-threshold

\[I = I_{D0} \frac{W}{L} e^{(V_{GS} - V_{th})/n U_{T}}\text{ ,} U_T = \frac{k T}{q}\]
\[I = \ell e^{V_{GS}/n U_{T}}\text{ , } \ell = I_{D0}\frac{W}{L} e^{-V_{th}/n U_{T}}\]
\[V_{GS} = n U_{T} \ln\left(\frac{I}{\ell}\right)\]

\[V_1 + V_2 = V_3 + V_4\]
\[n U_{T}\left[\ln\left(\frac{I_1}{\ell_1}\right) +
\ln\left(\frac{I_2}{\ell_2}\right)\right] = n
U_{T}\left[\ln\left(\frac{I_3}{\ell_3}\right) +
\ln\left(\frac{I_4}{\ell_4}\right)\right]\]
\[\ln\left(\frac{I_1 I_2}{\ell_1 \ell_2}\right) = \ln\left(\frac{I_3
I_4}{\ell_3 \ell_4}\right)\]
\[\frac{I_1 I_2}{\ell_1 \ell_2}= \frac{I_3
I_4}{\ell_3 \ell_4}\]
\[I_1 I_2 = I_3 I_4\text{ , if } \ell_1 \ell_2= \ell_3 \ell_4\]
\[I_1 I_2 = I_3 I_4\]
\[I_1 = I_a\text{ ,}I_2 = I_b + i_b\text{ ,}I_3 = I_b\text{ ,}I_4 = I_a + i_a\]
\[I_a (I_b + i_b) = I_b (I_a + i_a)\]
\[I_a I_b + I_a i_b = I_b I_a + I_b i_a\]
\[i_b = \frac{I_b}{I_a} i_a\]
\[\ell_1 \ell_2= \ell_3 \ell_4\]
\[\ell_1 = I_{D0}\frac{W}{L} e^{-V_{th}/n U_{T}}\]
\[\ell_2 = I_{D0}\frac{W}{L} e^{-(V_{th} \pm \sigma_{th})/n U_{T}} = \ell_1 e^{\pm \sigma_{th}/n U_{T}}\]
\[\sigma_{th} = \frac{a_{vt}}{\sqrt{W L}}\]
\[\frac{\ell_2}{\ell_1} = e^{\pm \frac{a_{vt}}{\sqrt{W L}}/n U_{T}}\]
Demo
JNW_SV_SKY130A
Want to learn more?
An Always-On 3.8 u J/86 % CIFAR-10 Mixed-Signal Binary CNN Processor With All Memory on Chip in 28-nm CMOS
CAP-RAM: A Charge-Domain In-Memory Computing 6T-SRAM for Accurate and Precision-Programmable CNN Inference
ARCHON: A 332.7TOPS/W 5b Variation-Tolerant Analog CNN Processor Featuring Analog Neuronal Computation Unit and Analog Memory
IMPACT: A 1-to-4b 813-TOPS/W 22-nm FD-SOI Compute-in-Memory CNN Accelerator Featuring a 4.2-POPS/W 146-TOPS/mm2 CIM-SRAM With Multi-Bit Analog Batch-Normalization